
Typically, this process is strenuous and affects the rate of efficiency. However, managing these partnerships calls for document-based communication. The ever-increasing, tough competition within various business industries is causing companies to form some form of alliance. It is needed for businesses that intend to execute a security strategy consistently across all applications, data, and networks. It is the concern of many IT experts to store more data at the edge of their networks, while also guaranteeing the security of the data. This is an important issue that validates the need for IT transformation. This, therefore, enables the business executives to concentrate on other business issues.
TRANSFORM IT MANUAL
It helps to develop automated processes that influence manual processes that affect payroll. It provides substantial solutions to important business operations such as finance and human resources. This is why IT transformation is essential. It is the ultimate objective of every business executive to maximize the productivity and effectiveness of the staff.
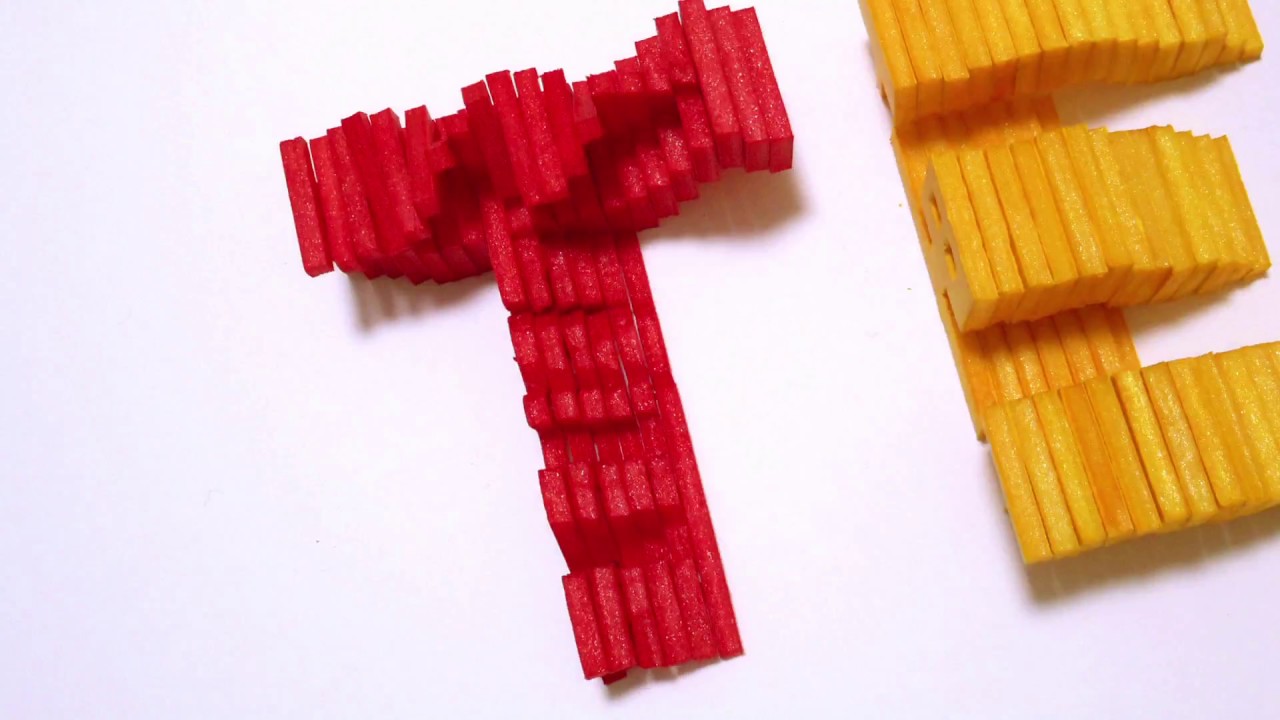
This is why businesses are demanding for more hybrid IT solutions that will guarantee the right customer experience. However, this can be quite difficult for a business to achieve.
TRANSFORM IT PROFESSIONAL
In today’s market, the larger proportion of customers desire to experience the exact kind of feeling in a professional setting that they get with the utilization of technology in their personal activities.
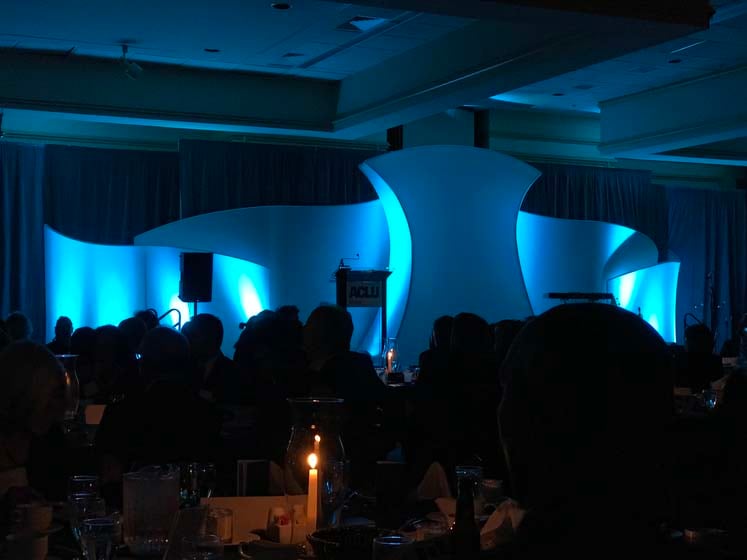
Attributes : scale_ ndarray of shape (n_features,) or None If True, scale the data to unit variance (or equivalently, Matrix which in common use cases is likely to be too large to fit in Sparse matrices, because centering them entails building a dense

This does not work (and will raise an exception) when attempted on Not a NumPy array or scipy.sparse CSR matrix, a copy may still be This is not guaranteed to always work inplace e.g. If False, try to avoid a copy and do inplace scaling instead. With_mean=False to avoid breaking the sparsity structure of the data.

This scaler can also be applied to sparse CSR or CSC matrices by passing Than others, it might dominate the objective function and make theĮstimator unable to learn from other features correctly as expected. If a feature has a variance that is orders of magnitude larger Machines or the L1 and L2 regularizers of linear models) assume thatĪll features are centered around 0 and have variance in the same Gaussian with 0 mean and unit variance).įor instance many elements used in the objective function ofĪ learning algorithm (such as the RBF kernel of Support Vector Individual features do not more or less look like standard normallyĭistributed data (e.g. Machine learning estimators: they might behave badly if the Standardization of a dataset is a common requirement for many Standard deviation are then stored to be used on later data using The relevant statistics on the samples in the training set. Where u is the mean of the training samples or zero if with_mean=False,Īnd s is the standard deviation of the training samples or one ifĬentering and scaling happen independently on each feature by computing
